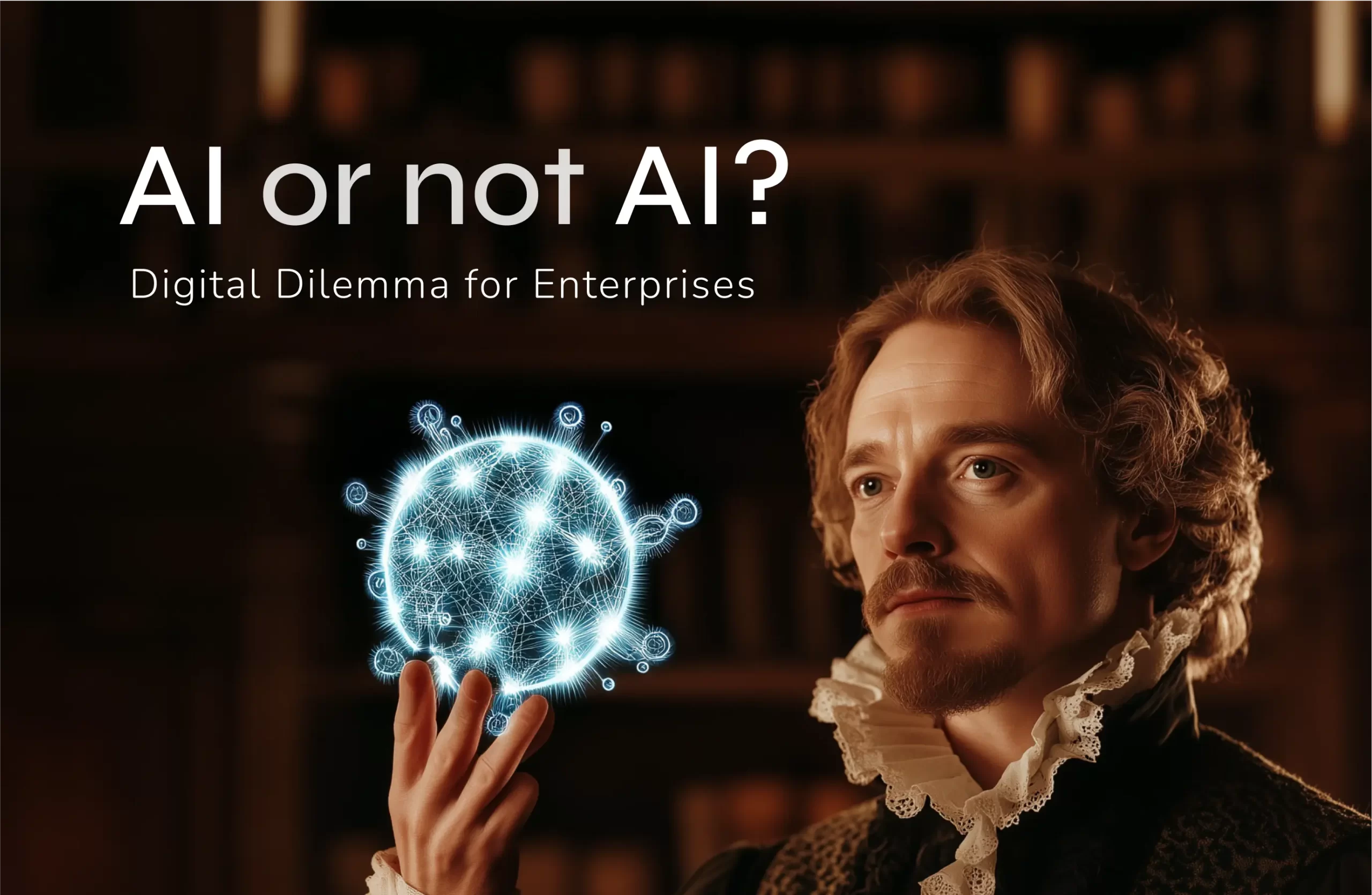
AI for Enterprises: Wait or Adopt despite Hallucinations?
An ongoing machine learning development fizzes speculative noise around large language models (LLM) and generative artificial intelligence (AI). Opinions about its implementation in business processes have been divided. IBM study shows that the share of AI adopters is 42%, while an additional 40% are actively exploring using the technology. On the other hand, there are skeptics who warn companies to refrain from using the technology.
What makes businesses avoid AI power? Mistrust. Four in five executives see at least one trust-related issue as a roadblock to generative AI adoption. One of the most widely discussed concerns is AI hallucinations. But is this really a valid justification for stealing the implementation of AI in business processes, given that it may cost the company dearly in the future?
Let’s figure it out.
What are AI hallucinations, and how harmful are they?
AI hallucinations are LMM-generated outputs that are false or inaccurate. When they sound absurd, they can do no harm. For example, in responding to the query “How many stones should a person eat?”, Google AI Overview suggested consuming one stone per day as they contain minerals useful for human health.
But there are cases when outputs sound appropriate, and without fact-checking, the user won’t suspect they are false. Such misleading results can be harmful and even cause serious losses.
In 2023, American lawyer Steven Schwartz used ChatGPT when preparing materials for one of the court cases. AI has generated several examples of similar court cases as a precedent base, but when the judge checked them, it proved that they never existed. As a result, the lawyer’s team was fined $5,000, not to mention the reputational damage he suffered.
Progress in combating AI hallucinations
Considering AI’s benefits to business, developers are putting much effort into eradicating hallucinations. There are dozens, if not hundreds, of solutions, I will list a few popular options.
Retrieval Augmented Generation (RAG): This approach involves supplementing LLM with information from external databases. A company can integrate its own corporate database or part of it into LLM to provide AI with more context for specific requests so that it can generate accurate answers.
Decoding by Contrasting Layers (DoLa). This is a technique for leveling AI hallucinations in pre-trained LLMs. Putting it simply, DoLa compares the old layers of information within the model with the new ones and correlates them. Such a comparative analysis allows the model to update the data, which reduces the possibility of generating inaccurate results on requests.
Decompose and Query Framework (D&Q). The next solution is the Decomposition and Query Framework. The essence can be conditionally divided into three key stages:
- Decomposition of the query: The user’s question is divided into several sub-questions, which are easier for LLM to understand and provide an answer to. After that, LLM applies a set of rules, such as compliance with the reliability of facts (consistency of facts) and relevance to the original question.
- Multi-level response: Next, LLM processes each sub-question. Starting from the second one, the model takes into account the information obtained from the answer to the previous sub-question.
- Concatenation and summarization: In the final step, all responses are summed up, and LLM generates a result.
Investments into next-level LLMs with built-in anti-hallucination remedies are gaining momentum and indicate that solving the problem is only a matter of time. For example, Microsoft invested $13 billion in OpenAI within the last five years. According to the company, their new AI model, known as a “Strawberry” project will hit a new milestone in reasoning and logical capacities (and more).
Looking at today’s realities and trends, I tend to think the creation of hybrid models will most likely remain the main direction in the fight against AI hallucinations.
The benefits of AI for enterprises are already here
While some companies are procrastinating with introducing AI into their business processes, the enterprises that have already deployed them have gained fundamental and strategic advantages.
According to McKinsey’s 2023 and 2024 research, AI can significantly reduce operating expenses and increase profits in enterprise-level companies due to the:
- Better efficiency;
- Acceleration of internal processes;
- Improving customer experience;
- Improvement of risk management;
- Better decision-making (interesting fact: On average, enterprise companies lose at least 3% of their profits because of low-quality decisions, translating into $150 million annually for a $5 billion company.)
Implementation of the technology allows companies to analyze business-critical data deeply in real-time. Therefore, they can quickly rebuild their commercial strategies if necessary.
The Price of The AI Adoption Delay
As of now, there is no alternative to AI power in its capability to process large amounts of data so quickly and thoroughly. There is no other tech option to automate and optimize business processes at all levels of enterprises, which are necessary for development today and prospering in the future. Therefore, the adoption of AI by enterprises is inevitable sooner or later.
When the problem of hallucinations is eventually minimized or completely vanished, today’s LLM skeptics will only start deploying AI infrastructure and retraining their specialists. Assume that such companies will need 2-4 years to adapt to the new technological context.
At the same time, today’s AI adopters will already have full-fledged business processes aboard by then.
They’ve configured the technical infrastructure, installed the necessary software, and provided access to the appropriate services for users or employees. They’ve trained their workforce and shaped a pool of specialists with AI-savvy talents, who will become increasingly in-demand and, therefore, difficult and expensive to hire.
Those companies will be way ahead of their competitors because they will be able to implement all innovations and disruptions in AI without delay. Eventually, such enterprises will be forward-looking and strategic businesses.
Best regards,
Author: Vitaliy Goncharuk
12New.AI / BestAgreement.ai
CEO & Founder