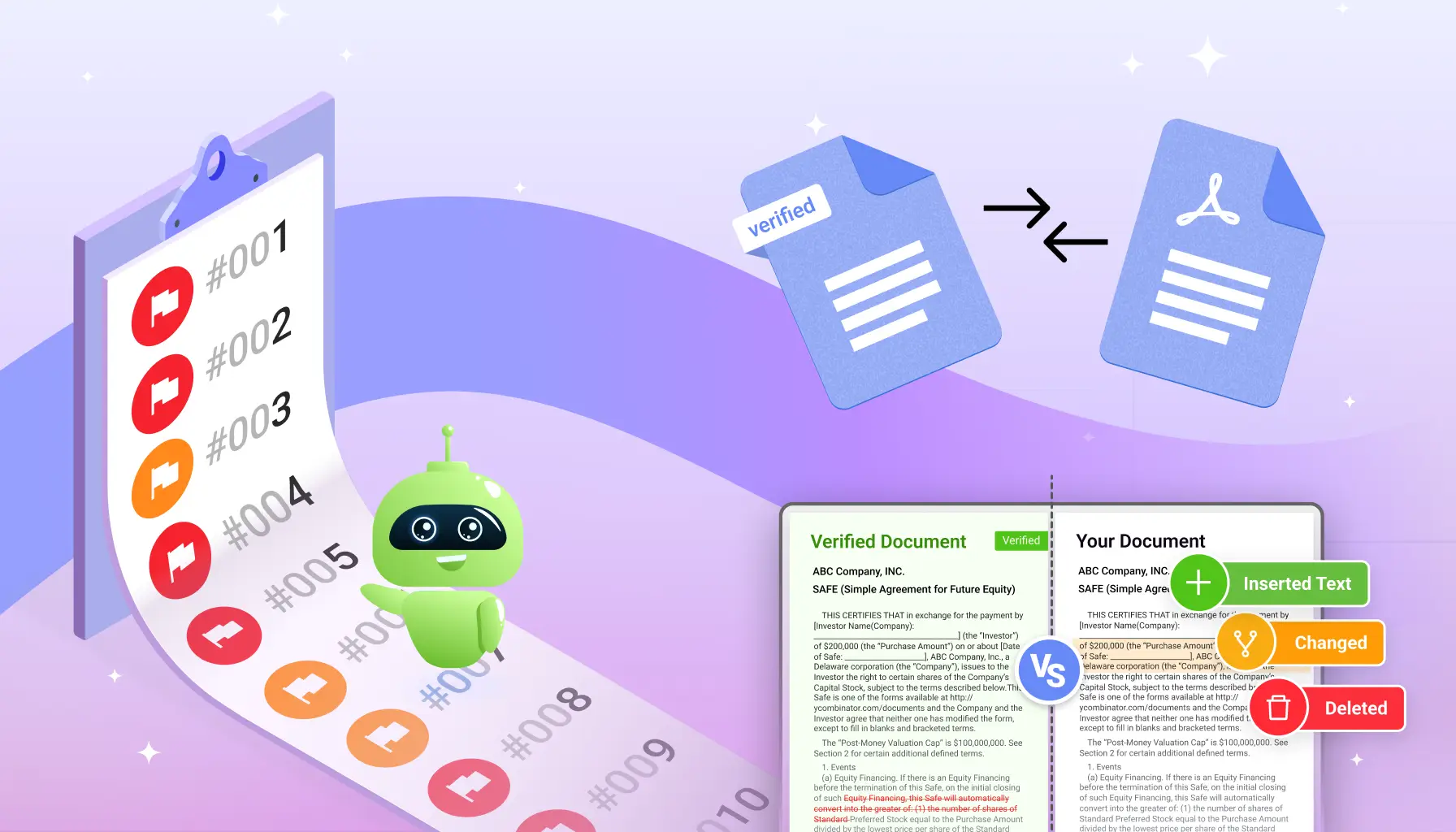
From Proprietary to Open-Source AI: Where Enterprises Are Shifting?
Open-source AI versus closed-source AI has become a topic of fierce debate. On the one hand, tech is rapidly democratizing due to open source, but on the other hand, security and profits remain two major concerns for enterprises regarding AI adoption.
As I personally observe, and recent research backs up those observations, the explosive growth of generative AI is transforming enterprises’ approaches to adopting technologies.
In this article, I’d like to share my thoughts on this topic.
Open-Source AI vs. Closed-Source AI
In traditional software development, the distinction between open-source and closed-source focuses on restrictions around code reuse. The difference in AI is more complicated. Except for the code’s openness, which determines the opportunity to inspect, modify, and improve it, there is also the model (training process and proprietary information) and data (where it came from, how it was collected and cleaned, and what it consists of). Each part can be open or closed.
Many organizations adopt a mixed approach, sharing some elements openly while keeping others closed. For example, an enterprise might open-source its code but keep its training data and the specifics of its model architecture private.
But let’s delve into the main aspects that determine the attractiveness of a particular approach for enterprises.
Closed AI: Benefits and Examples
Closed AI, also referred to as “proprietary,” is basically a private property that can be licensed. This means owners can monetize their investments in the product simply by selling it. Competitors cannot access the code, architecture, and data and, therefore, can’t replicate and sell it (or give it away for free) themselves.
Selling means responsibility for the quality. Closed AI is usually developed with proper monitoring and rigorous governance, which helps to avoid biased and improper outcomes common with open-source algorithms.
The other important thing is security. Vendors must protect their models from data leaks or unauthorized access; otherwise, they may face reputational damage and legal issues.
Examples of closed-source generative AI are Google’s Gemini, Midjourney, Nvidia Jarvis, GPT-4, and Dall-E (OpenAI is closed, ironically).
Open-source AI: Benefits and Examples
Open-source lacks such luxury as monetization, but their deployment requires much less investment because businesses can build solutions based on already created architecture without the licensing fees.
However, the most crucial benefit of open-source AI–and most of the experts are unanimous in this matter–is its accessibility to the vast community. Collective innovation accelerates scalability. The power of community helps to identify and mitigate problems better and brings transparency and peer review to the table.
Examples of open-source generative AI: Stable Diffusion, Meta’s Llama, TensorFlow, PyTorch, and Hugging Face.
And what about drawbacks? While Open-sourced AI is more vulnerable to so-called hallucinations and regulators’ concerns, its Closed-sourced peer is expensive and limited in terms of scaling.
Let’s see what options enterprises choose, considering all of the factors described above.
Enterprises’ Preferences: Open-Source AI is Gaining Momentum
A recent report by Andreessen Horowitz has shown shifting toward greater use of open-source AI among businesses. The analytics have spoken with dozens of Fortune 500 and top enterprise leaders and surveyed 70 more, to understand how they’re using, buying, and budgeting for generative AI. As a result, they’ve revealed significant changes in attitudes toward genAI, particularly in choosing between open-sourced and closed-sourced models.
While in 2023, Andreessen Horowitz estimated the market share was 80%–90% closed source, in 2024, 46% of respondents mentioned that they prefer or strongly prefer open source models.
Nearly 60% of AI leaders noted that they were interested in increasing open source usage or switching when fine-tuned open source models roughly matched the performance of closed-source models.
In 2024 and onwards, enterprises expect a significant shift of usage towards open source, with some expressly targeting a 50/50 split—up from the 80% closed/20% open split in 2023.
Open-source AI models are appealing for businesses due to their customizability (ability to fine-tune for a given use case effectively), control (security of proprietary data and understanding why models produce certain outputs), and cost-effectiveness which surprisingly for analytics wasn’t top of mind.
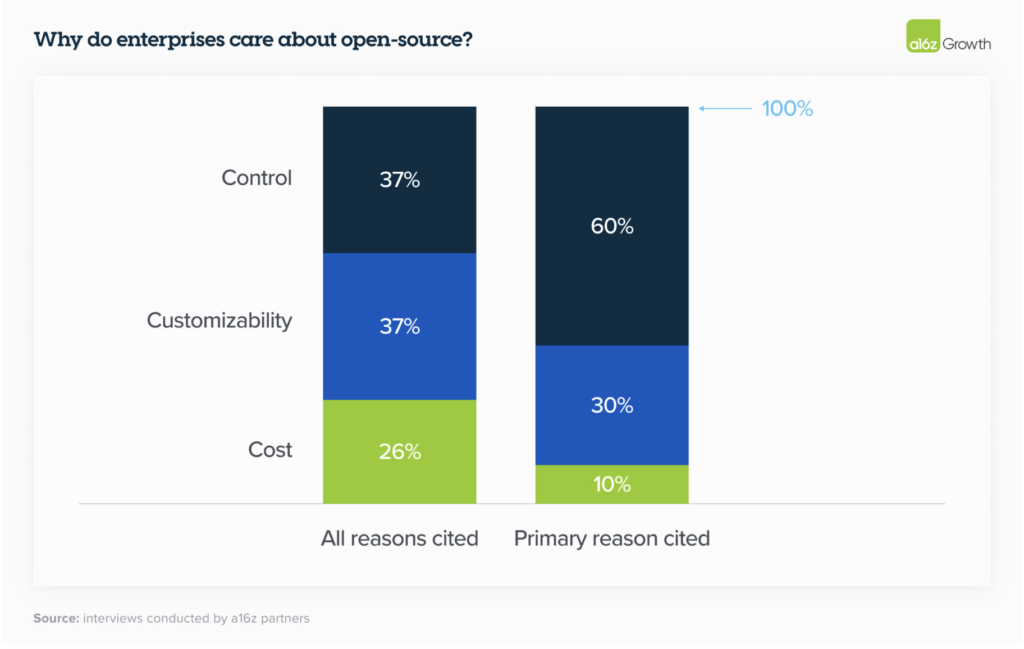
Open-Sourced Models Drive the Future of GenAI
I think open-source platforms are more promising than closed-source platforms in the medium and long term. Further development of AI will be driven by open principles because novelty is unlikely to happen in a closed scenario. Technological democratization, intrinsic to open-source, empowers a large population of developers for rapid innovation.
Cooperation leads to advanced AI capabilities and their wider availability. I am currently observing a new wave of AI agents emerging with a lot of open source within. I anticipate that open-source models will eventually overcome drawbacks like hallucinations and bias and will become killers for closed-source solutions.
Best regards,
Author: Vitaliy Goncharuk
12New.AI / BestAgreement.ai
CEO & Founder